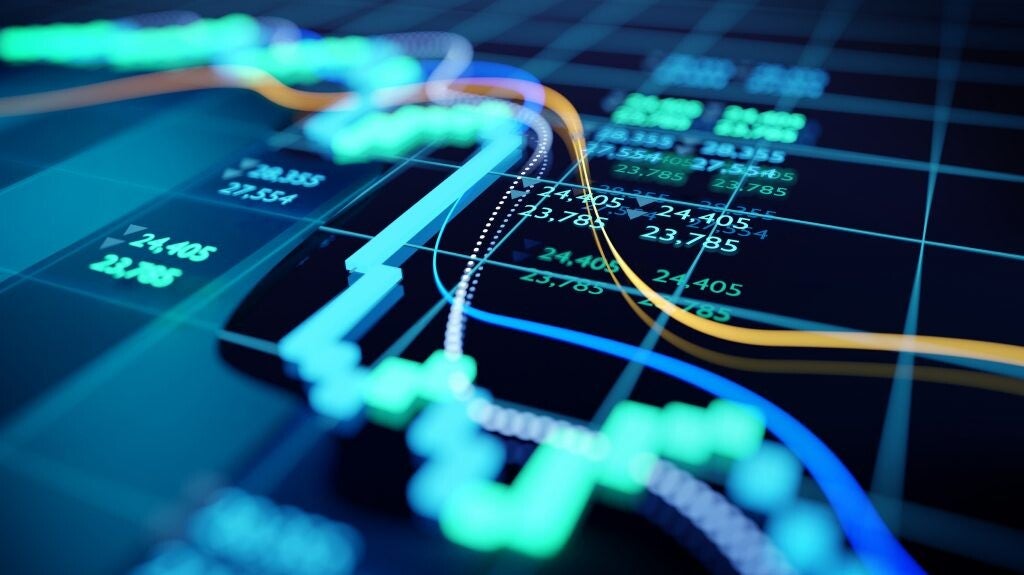
Lachlan McCalman and Daniel Steinberg , Gradient Institute
Grace Abuhamad and Marc-Etienne Brunet , ServiceNow
Robert C. Williamson , University of Tübingen
Richard Zemel, University of Toronto
If society demands that a bank’s use of artificial intelligence systems is
“fair,” what is the bank to actually do?
This article outlines a pragmatic and defensible answer.
Artificial intelligence (AI) systems are becoming ubiquitous in a diverse and ever-growing set of decision-making applications, including in the financial sector. AI systems can make consequential decisions at a speed and volume
not possible for humans, creating new opportunities to improve and personalize customer service but also increasing the scale of potential harm they can cause if they are misdesigned.
AI systems unfairly discriminating against individuals by their race, gender, or
other attributes is a particularly common and disheartening example of this harm. For example, soon after l aunching its credit card partnership with Goldman Sachs in 2019, Apple had to investigate its system for gender bias. This bias, if left unchecked, could have limited women’s access to credit,
harming those potential customers and increasing risks of regulatory noncompliance for the business.
However, there is no simple solution to preventing these kinds of incidents: helping AI live up to its promise of better and fairer decision making is a tremendous technical and social challenge. One of the key design mistakes behind harmful AI systems in use today is an absence of explicit and precise ethical objectives or constraints. Unlike humans, AI systems cannot apply even a basic level of moral awareness to their decision making by default. Only by encoding mathematically precise statements of our ethical standards into our designs can we expect AI systems to meet those standards.
Technical work to develop such ethical encodings is burgeoning, with much of the focus on the fairness of AI systems in particular. This work typically involves developing mathematically precise measures of fairness suitable for designing into AI systems. Fairness measures use the system’s data, predictions, and decisions to characterize its fairness according to a specific definition (for example, by comparing the error rates of the system’s predictions between men and women). The exercise of defining fairness in mathematical terms has not “solved” fairness but rather surfaced the complexity of the problem at the definitional stage. There now exists a panoply of fairness measures, each corresponding to a different notion of fairness and potentially applicable in different contexts.
Add comment
Comments