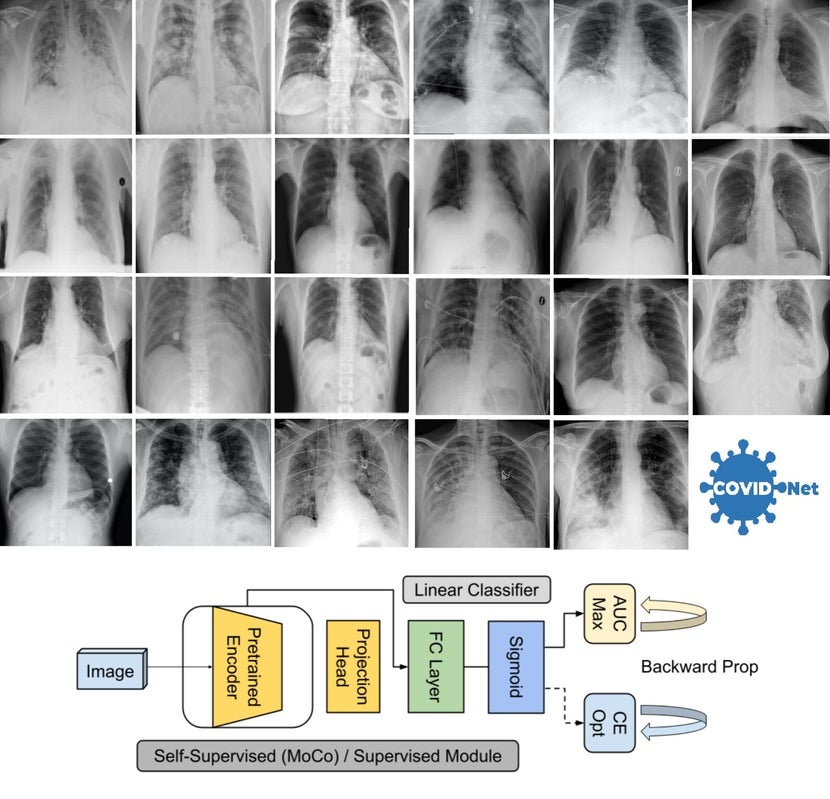
Siyuan He, Pengcheng Xi, Ashkan Ebadi, Stephane Tremblay, Alexander Wong
Effective representation learning is the key in improving model performance for medical image analysis. In training deep learning models, a compromise often must be made between performance and trust, both of which are essential for medical applications. Moreover, models optimized with cross-entropy loss tend to suffer from unwarranted overconfidence in the majority class and over-cautiousness in the minority class. In this work, we integrate a new surrogate loss with self-supervised learning for computer-aided screening of COVID-19 patients using radiography images. In addition, we adopt a new quantification score to measure a model's trustworthiness. Ablation study is conducted for both the performance and the trust on feature learning methods and loss functions. Comparisons show that leveraging the new surrogate loss on self-supervised models can produce label-efficient networks that are both high-performing and trustworthy.
Add comment
Comments