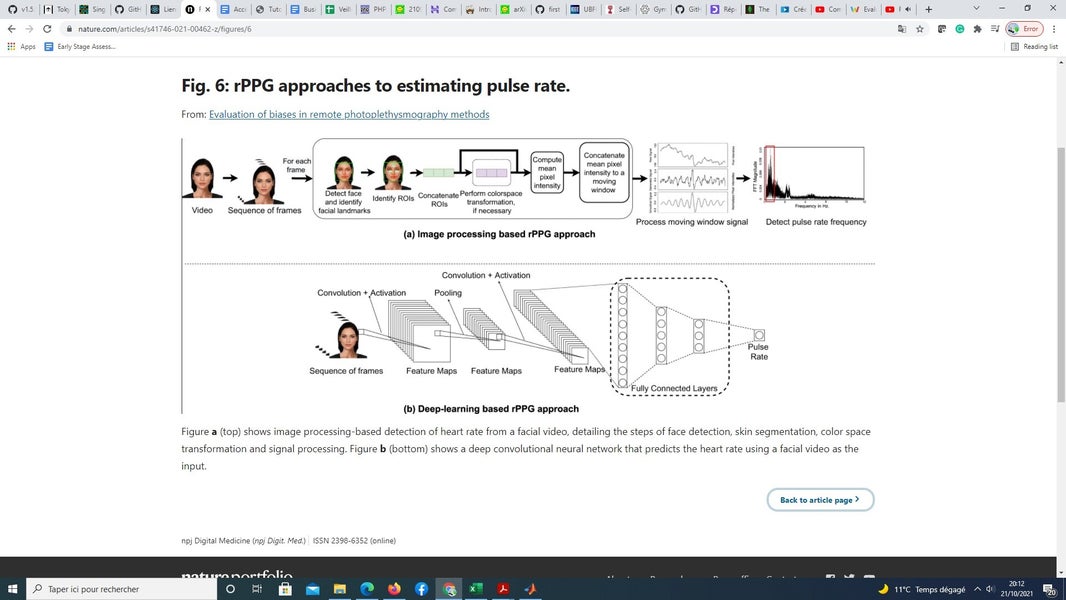
Abstract
This work investigates the estimation biases of remote photoplethysmography (rPPG) methods for pulse rate measurement across diverse demographics. Advances in photoplethysmography (PPG) and rPPG methods have enabled the development of contact and noncontact approaches for continuous monitoring and collection of patient health data. The contagious nature of viruses such as COVID-19 warrants noncontact methods for physiological signal estimation. However, these approaches are subject to estimation biases due to variations in environmental conditions and subject demographics. The performance of contact-based wearable sensors has been evaluated, using off-the-shelf devices across demographics. However, the measurement uncertainty of rPPG methods that estimate pulse rate has not been sufficiently tested across diverse demographic populations or environments. Quantifying the efficacy of rPPG methods in real-world conditions is critical in determining their potential viability as health monitoring solutions. Currently, publicly available face datasets accompanied by physiological measurements are typically captured in controlled laboratory settings, lacking diversity in subject skin tones, age, and cultural artifacts (e.g, bindi worn by Indian women). In this study, we collect pulse rate and facial video data from human subjects in India and Sierra Leone, in order to quantify the uncertainty in noncontact pulse rate estimation methods. The video data are used to estimate pulse rate using state-of-the-art rPPG camera-based methods, and compared against ground truth measurements captured using an FDA-approved contact-based pulse rate measurement device. Our study reveals that rPPG methods exhibit similar biases when compared with a contact-based device across demographic groups and environmental conditions. The mean difference between pulse rates measured by rPPG methods and the ground truth is found to be ~2% (1 beats per minute (b.p.m.)), signifying agreement of rPPG methods with the ground truth. We also find that rPPG methods show pulse rate variability of ~15% (11 b.p.m.), as compared to the ground truth. We investigate factors impacting rPPG methods and discuss solutions aimed at mitigating variance.
Introduction
Changes in physiological signals of the human body, such as pulse rate, body temperature, blood pressure, and respiration rate can be monitored using invasive (sensors are inserted into the body) or noninvasive (sensors are not inserted into the body) methods1. Noninvasive methods are further classified as contact (sensor makes contact with the skin) and noncontact (take measurements from a distance) methods2. Contact-based methods monitor physiological signals by measuring changes in physical properties, such as pressure, temperature, and light transmitted/reflected3. Noncontact physiological signal monitoring is primarily achieved using camera, audio, infrared (IR), ultrasound, or Doppler-based approaches (Advanced Non-contact Patient Monitoring Technologies: A New Paradigm in Healthcare Monitoring), each differing in the type of input signal used for measurement. These approaches have gained momentum for remote monitoring of physiological signals of subjects (Advanced Non-contact Patient Monitoring Technologies: A New Paradigm in Healthcare Monitoring). Of these methods, video modality has become the predominant input data type for noncontact approaches, driven by the availability of low-cost cameras and smartphones4. The COVID-19 pandemic has also contributed to increased usage of noncontact technology for monitoring vital signs of patients, as well as analyzing the effects of new drugs5. Remote photoplethysmography (rPPG) is a noncontact video-based method that monitors the change in blood volume by capturing pixel intensity changes from the skin to measure pulse rate6. In contrast, contact-based photoplethysmography (PPG) sensors emit light onto the skin and measure the pulse rate by detecting the amount of light transmitted or reflected (i.e., Skin reflection model7). PPG approaches may not be suitable for vital sign measurements in situations involving contagious diseases (e.g., COVID-19), due to the need for physical contact and sterilization procedures after use8. rPPG approaches make no physical contact with the person whose physiological measurements are being captured, making them suitable for situations necessitating noncontact approaches. Moreover, rPPG methods have the potential to be integrated into existing mobile phones (e.g., via an app download), especially given the increased prevalence of mobile devices in resource constrained environments4.
The differences between estimated and actual physiological signals can be due to multiple sources of noise9,10. Sensor noise varies with the type of sensor, conditions during measurement, human error, and bias due to subject demographics9. The measurement noise for PPG methods across subject demographics has been studied in detail by Bent et al.11. The quantification of rPPG measurement biases across demographics remains an open area of scientific inquiry. The primary objective of this work is to investigate sources of error across state-of-the-art rPPG methods and an FDA-approved gold standard pulse rate measurement device in demographic populations typically not represented in evaluation datasets. We investigate factors responsible for causing pulse rate estimation bias, while using state-of-the-art rPPG methods on facial videos.
Commonly used datasets for tasks related to processing facial videos, such as BP4D and Multi-Pie, are unbalanced in terms of demographic diversity12,13. A majority of subjects are from Euro-American (49%) descent followed by Asians (27%) in the age group of 19–29 years. Other publicly available datasets, such as MAHNOB-HCI and MMSE-HR, also show similar demographic composition14. These datasets are also collected under optimal illumination conditions in controlled laboratory settings. In contrast, the dataset used for this study is collected from two geographically and culturally distinct countries, India and Sierra Leone, introducing environmental and subject variations that are typically underrepresented in publicly available face datasets. Increasing the diversity of datasets is critical to minimizing algorithmic biases. Further, the demographic representation in the dataset used in this study contains subjects with features, such as facial marks or adornments and headgear (Fig. 1), which are typically not represented in existing datasets used to train or evaluate rPPG methods. For example, the presence of facial marks, such as a “bindi” worn by Indian women, may interfere with spatial pixel averaging in the forehead skin region of interest and may cause biased estimation of pulse rate. The presence of headgear, such as hats or turbans, may cause occlusion leading to errors in face detection. A knowledge gap exists in terms of how well existing state-of-the-art rPPG methods perform when presented with diverse datasets. This work
-
Performs data collection of facial videos of subjects containing demographics typically not represented in existing datasets used to evaluate rPPG methods for pulse rate estimation.
-
Compares and tests the estimation accuracy of state-of-the-art rPPG methods against an FDA-approved ground truth PPG sensor on the collected demographically diverse dataset.
-
Investigates the sources of bias in rPPG approaches for pulse rate estimation, such as country of origin, gender, and skin tone, and quantifies the error due to each identified
Add comment
Comments